In brief
- Tiny, open-source AI model Dia-1.6B claims to beat industry giants like ElevenLabs or Sesame at emotional speech synthesis.
- Creating convincing emotional AI speech remains challenging due to the complexity of human emotions and technical limitations.
- While it matches up well against competition, the “uncanny valley” problem persists as AI voices sound human but fail at conveying nuanced emotions.
Nari Labs has released Dia-1.6B, an open-source text-to-speech model that claims to outperform established players like ElevenLabs and Sesame in generating emotionally expressive speech. The model is super tiny—with just 1.6 billion parameters—but still can create realistic dialogue complete with laughter, coughs, and emotional inflections.
It can even scream in terror.
We just solved text-to-speech AI.
This model can simulate perfect emotion, screaming and show genuine alarm.
— clearly beats 11 labs and Sesame
— it’s only 1.6B params
— streams realtime on 1 GPU
— made by a 1.5 person team in Korea!!It’s called Dia by Nari Labs. pic.twitter.com/rpeZ5lOe9z
— Deedy (@deedydas) April 22, 2025
While that might not sound like a huge technical feat, even OpenAI’s ChatGPT is flummoxed by that: “I can’t scream but I can definitely speak up,” its chatbot replied when asked.
Now, some AI models can scream, if you ask them to. But it’s not something that happens naturally or organically, which, apparently, is Dia-1.6B’s super power. It understands that, in certain situations, a scream is appropriate.
Nari’s model runs in real-time on a single GPU with 10GB of VRAM, processing about 40 tokens per second on an Nvidia A4000. Unlike larger closed-source alternatives, Dia-1.6B is freely available under the Apache 2.0 license through Hugging Face and GitHub repositories.
“One ridiculous goal: build a TTS model that rivals NotebookLM Podcast, ElevenLabs Studio, and Sesame CSM. Somehow we pulled it off,” Nari Labs co-founder Toby Kim posted on X when announcing the model. Side-by-side comparisons show Dia handling standard dialogue and nonverbal expressions better than competitors, which often flatten delivery or skip nonverbal tags entirely.
The race to make emotional AI
AI platforms are increasingly focused on making their text-to-speech models show emotion, addressing a missing element in human-machine interaction. However, they are not perfect and most of the models—open or closed—tend to create an uncanny valley effect that diminishes user experience.
We have tried and compared a few different platforms that focus on this specific topic of emotional speech, and most of them are pretty good as long as users get into the right mindset and know their limitations. However, the technology is still far from convincing.
To tackle this problem, researchers are employing various techniques. Some train models on datasets with emotional labels, allowing AI to learn the acoustic patterns associated with different emotional states. Others use deep neural networks and large language models to analyze contextual cues for generating appropriate emotional tones.
ElevenLabs, one of the market leaders, tries to interpret emotional context directly from text input, looking at linguistic cues, sentence structure, and punctuation to infer the appropriate emotional tone. Its flagship model, Eleven Multilingual v2, is known for its rich emotional expression across 29 languages.
Meanwhile, OpenAI recently launched “gpt-4o-mini-tts” with customizable emotional expression. During demonstrations, the firm highlighted the ability to specify emotions like “apologetic” for customer support scenarios, pricing the service at 1.5 cents per minute to make it accessible for developers. Its state of the art Advanced Voice mode is good at mimicking human emotion, but is so exaggerated and enthusiastic that it could not compete in our tests against other alternatives like Hume.
Where Dia-1.6B potentially breaks new ground is in how it handles nonverbal communications. The model can synthesize laughter, coughing, and throat clearing when triggered by specific text cues like “(laughs)” or “(coughs)”—adding a layer of realism often missing in standard TTS outputs.
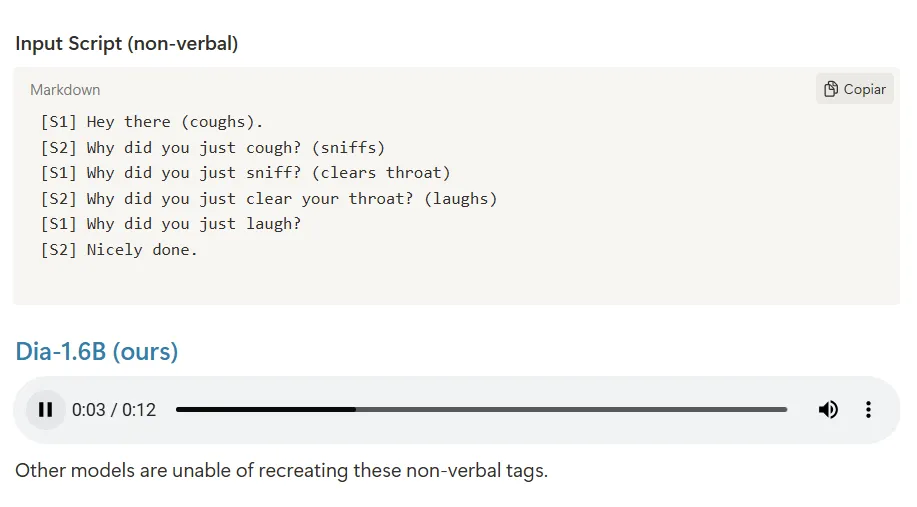
Beyond Dia-1.6B, other notable open-source projects include EmotiVoice—a multi-voice TTS engine that supports emotion as a controllable style factor—and Orpheus, known for ultra-low latency and lifelike emotional expression.
It’s hard to be human
But why is emotional speech so hard? After all, AI models stopped sounding robotic a long time ago.
Well, it seems like naturality and emotionality are two different beasts. A model can sound human and have a fluid, convincing tone, but completely fail at conveying emotion beyond simple narration.
“In my view, emotional speech synthesis is hard because the data it relies on lacks emotional granularity. Most training datasets capture speech that is clean and intelligible, but not deeply expressive,” Kaveh Vahdat, CEO of the AI video generation company RiseAngle, told Decrypt. “Emotion is not just tone or volume; it is context, pacing, tension, and hesitation. These features are often implicit, and rarely labeled in a way machines can learn from.”
“Even when emotion tags are used, they tend to flatten the complexity of real human affect into broad categories like ‘happy’ or ‘angry’, which is far from how emotion actually works in speech,” Vahdat argued.
We tried Dia, and it is actually good enough. It generated around one second of audio per second of inference, and it does convey tonal emotions, but is so exaggerated that it doesn’t feel natural. And this is the key of the whole problem—models lack so much contextual awareness that it is hard to isolate a single emotion without additional cues and make it coherent enough for humans to actually believe it is part of a natural interaction
The “uncanny valley” effect poses a particular challenge, as synthetic speech cannot compensate for a neutral robotic voice simply by adopting a more emotional tone.
And there are more technical hurdles abound. AI systems often perform poorly when tested on speakers not included in their training data, an issue known as low classification accuracy in speaker-independent experiments. Real-time processing of emotional speech requires substantial computational power, limiting deployment on consumer devices.
Data quality and bias also present significant obstacles. Training AI for emotional speech requires large, diverse datasets capturing emotions across demographics, languages, and contexts. Systems trained on specific groups may underperform with others—for instance, AI trained primarily on Caucasian speech patterns might struggle with other demographics.
Perhaps most fundamentally, some researchers argue that AI cannot truly mimic human emotion due to its lack of consciousness. While AI can simulate emotions based on patterns, it lacks the lived experience and empathy that humans bring to emotional interactions.
Guess being human is harder than it seems. Sorry, ChatGPT.
Generally Intelligent Newsletter
A weekly AI journey narrated by Gen, a generative AI model.